Uncertainty Estimation in Text Generation: A New Metric for Language Models (LLMs)
Rhythm Blues AI - Un pódcast de Andrea Viliotti, digital innovation consultant (augmented edition)
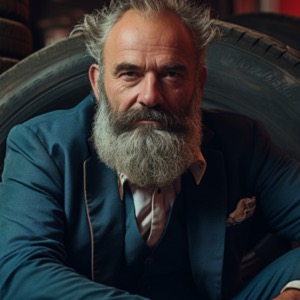
The episode delves into the study titled "Rethinking Uncertainty Estimation in Natural Language Generation," which introduces G-NLL, an innovative metric for estimating uncertainty in text generation by large language models (LLMs). Unlike traditional metrics that require multiple sampling, G-NLL relies on the probability of the most likely sequence, significantly reducing computational costs. The research demonstrates the effectiveness of G-NLL through empirical tests conducted on various datasets and models, showing competitive performance compared to existing metrics, particularly for shorter text generations. This approach is especially advantageous for companies using LLMs in operational settings, providing a fast and efficient method to assess the reliability of generated responses. The simplicity of G-NLL makes it a strategic tool for improving risk management and optimizing resources, offering a practical solution to address operational challenges associated with the use of language models.