PROCESSBENCH: Toward a Scalable Evaluation of Mathematical Reasoning Errors in AI
Rhythm Blues AI - Un pódcast de Andrea Viliotti, digital innovation consultant (augmented edition)
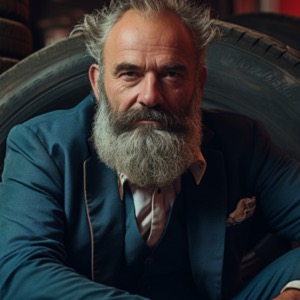
The episode examines the "PROCESSBENCH" study, which introduces an innovative method to evaluate the ability of language models to detect errors in step-by-step mathematical reasoning. This approach focuses on the entire logical process rather than just the final result. The study leverages a large dataset of 3,400 mathematical problems, ranging from school-level exercises to olympiad-level challenges, to compare two types of models: "process reward models," which reward only the correct answer, and "critic models," which are more flexible and capable of critical analysis. The findings reveal that "critic models" excel in identifying errors, even in highly complex problems, highlighting the importance of deeper approaches to assessing the reliability of automated reasoning systems. PROCESSBENCH aims to enhance transparency and robustness in the development of these technologies, offering valuable insights for the future regulation of the field.