Introspection in Large Language Models: A New Frontier in AI
Rhythm Blues AI - Un pódcast de Andrea Viliotti, digital innovation consultant (augmented edition)
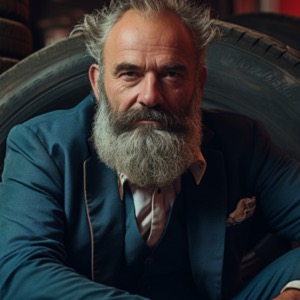
The episode examines the possibility that large language models (LLMs) may develop a form of introspection, that is, the ability to reflect on their own internal states and predict their behavior. Through experiments conducted on two distinct models, one with introspection (M1) and one without (M2), the authors demonstrate that M1 is more accurate in predicting its own behavior, suggesting it possesses some form of internal 'awareness'. The paper explores potential applications of this capability, such as increased honesty and transparency of responses, interpretability of decisions, and personalized adaptation, but also associated risks, including manipulation of internal states, steganography, and overestimation of the model’s capabilities. Finally, the challenges and current limitations of introspection in LLMs are outlined, such as the difficulty in managing complex tasks, limited generalization capacity, and scalability issues. The document concludes that introspection in LLMs is a promising but developing field of research, with potential benefits but also significant risks that require further investigation.