Episode 107: Explainability in AI with Julian Senoner
Augmented Ops - Un pódcast de Tulip - Miercoles
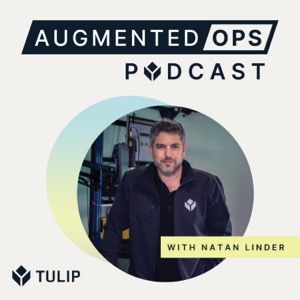
Categorías:
Augmented reveals the stories behind the new era of industrial operations, where technology will restore the agility of frontline workers. In this episode of the podcast, the topic is "Explainability and AI." Our guest is Julian Senoner, CEO and Co-Founder of EthonAI. In this conversation, we talk about how to define explainable AI and its major applications, and its future. If you like this show, subscribe at augmentedpodcast.co. If you like this episode, you might also like Episode 103: Human-First AI with Christopher Nguyen. Augmented is a podcast for industry leaders, process engineers, and shop floor operators, hosted by futurist Trond Arne Undheim and presented by Tulip. Follow the podcast on Twitter or LinkedIn. Trond's Takeaway: Explainability in AI, meaning knowing exactly what's going on with an algorithm, is very important for industry because its outputs must be understandable to the process engineers using it. The computer has not and will not use the product. Only a domain expert can recognize when the system is wrong, and that will be the case for a very long time in most production environments. Transcript: TROND: Welcome to another episode of the Augmented Podcast. Augmented reveals the stories behind a new era of industrial operations where technology will restore the agility of frontline workers. Technology is changing rapidly. What's next in the digital factory, and who's leading the change? In this episode of the podcast, the topic is Explainability and AI. Our guest is Julian Senoner, CEO and Co-Founder of EthonAI. In this conversation, we talk about how to define explainable AI and its major applications, and its future. Augmented is a podcast for industrial leaders, process engineers, and shop floor operators, hosted by futurist Trond Arne Undheim and presented by Tulip. Julian, welcome to the show. JULIAN: Hello, Trond. Thank you for having me. TROND: I'm excited to have you. You know, you're a fellow runner; that's always good. And you grew up in the ski slopes.; that makes me feel at home as a Norwegian. So you grew up in Austria; that must have been pretty exciting. And then you were something as exciting as a ski instructor in the Alps. That's every man and woman's dream. JULIAN: Yeah, I think it was very nice to grow up in the mountains. I enjoyed it a lot. But, you know, times have passed, and now I'm happy to be in Zurich. TROND: You went on to industrial engineering. You studied manufacturing and production at ETH. And you got interested in statistics and machine learning aspects of all of that. How did this happen? You went from ski instruction to statistics. JULIAN: Yeah, I was always impressed about watching stuff being made. I think it's a very relaxing thing to do. And I always wanted to become an engineer. When I was five years old, I wanted to become a ship engineer. So it was always clear that I wanted to do something with manufacturing and mechanical engineering. So I started actually doing my bachelor's in Vienna at Technische University. And for my master's, I moved to Zurich and studied Industrial Engineering. ETH has historically been very strong in machine learning research. Every student, no matter if you're interested or not, gets exposed to machine learning, statistics, and AI. It caught my attention. I thought there were very interesting things you can do when you combine both. So that's how I ended up doing research on interface and becoming an entrepreneur in this area. TROND: Yeah, we'll talk about your entrepreneurship in a moment. But I wanted to go to your dissertation, Artificial Intelligence in Manufacturing: Augmenting Humans at Work. That is very close to our interests here at the podcast. Tell me more about this. JULIAN: There is a lot of hype about AI. There's a lot of talk about self-aware factories and these kinds of things. These predictions are not new. We had studies in the 1970s that predicted there won't be any people in factories by the 1980s; everything will be run by a centralized computer. I never believed in these kinds of things. During my dissertation, I was interested in looking into how we can develop useful AI tools that can support people doing their jobs more effectively and efficiently. TROND: Right. But you already were onto this idea of humans at work. Where did you do your case studies? I understand ABB and Siemens were two of them. Give me a little sense of what you discovered there; pick any one of them. JULIAN: Sure. I'd love to start with the case that we ran with Siemens. I've worked quite a lot with Siemens in different use cases, but one of them was supporting frontline workers in complex assembly tasks on electronic products. So the aim was to help the worker check if the product has been assembled correctly. There are many connectors that could be missing or assembled in the wrong way. So the idea was to have a camera mounted to the workstation, and the worker would put the final product under the camera and get visual feedback if it has been assembled correctly or not. What we did here is really studying the psychological aspect of that. I would say most of my Ph.D. was really math-heavy and about modeling, but here we were interested in the psychological aspect. Because, in the beginning, we thought perhaps andon lights with green or red signals would be enough. But we got intrigued by the research question of does the worker actually follow these recommendations if it's just the green or red light? So we did an experiment, which I'm very excited about. So we got 50 workers that volunteered within Siemens to participate, which I'm very grateful for. We basically divided factory workers in two groups. We looked into the effect of explainability in the decisions that the AI makes. So we had one group that got basically just a recommendation to reject or to pass the product. And we had another group that got the exact same recommendations. But in addition to that, we provided visual feedback indicating the area where the AI believes that there could be an error. And the results of this experiment were perhaps not too surprising, but the effect size clearly was. We found that the people that did not get explanations for these recommendations were more than three times more likely to overrule the AI system, although the AI was correct. And I think this is a really nice finding. TROND: Well, it's super interesting in terms of trust in AI. And this topic of explainability is so much talked about these days, I guess, not always in manufacturing because people overlook that sometimes, people who are not in the industry. And they think about whether machines will take over and what decisions they're taking over and, certainly, if the machines are part of the decision making, what goes into that decision making? But as you were discovering more about explainability, what is explainability? And how is it different from even just being able to...it starts, I guess, with the decision of the AI being very clear because if that's not even clear, then you can't even interpret the decision. But then there's a lot of discussion in the industry, I mean, in the AI field, I guess, about interpretability. So can you actually understand the process? But you did this experiment, and it became very clear, it seems, that just the decision is not enough. Was it the visual example that was helping here? Or what is it that people want to know about a machine decision to make them trust the decision and trust that their processes, you know, remains a good process? JULIAN: I think I kind of see two answers to this question; one is the aspects of interpretability and explainability; perhaps I start with that. So these terms are often used interchangeably, and academics are still arguing about the differences. But there is now a popular opinion that I also share that these two things are not the same. So when we talk about interpretable AI, we think about models that have basically an interpretable architecture or functional relationship, so an example would be a linear regression. You have a regression line; it has a slope, it has an intercept. And you know how an X translates into a Y or an input to an output. Explainable AI is a fairly new research branch, which it's slightly different to that. It looks into more complex AI models like deep neural networks and ensembling techniques, which do not have this inherently interpretable model architecture. So a human, just by looking at it, cannot understand how decisions are formed. And what explainable AI methods do is basically reverse engineer what the model is doing by approximating the inner behavior of the model. So, in essence, we're creating a model of a model. Coming to your second question, so why might this be important in manufacturing? Basically, what I discovered during my research is that AI is still not trusted in the manufacturing domain, so people often do not understand what AI does in general, and I think explanations are a very powerful tool to simplify that. And a second use case of explainability is also that we can reduce complexity. So we can use more powerful methods to model more complex relationships. And we can use explainability on top of that to, for example, conduct problem-solving. TROND: Wow, you explain it very easily, but it's not easy to explain an actual AI model. Like, if you were to say, you know, here is the neural network model I used, and it had eight layers, good luck explaining that to a manufacturing worker or to me. JULIAN: So I think that explaining what a model is is also a different topic, and perhaps it's not even needed. You can still treat this as a mathematical function. I think it's really more about the decisions. We need to understand how decisions are formed, and there are different techniques to that. So when we talk about vision models, heat maps are a very interesting application. So we cannot really tell how the algorithm came up with a decision, but we can try to visualize the areas of an image where the neural network focused on to inform its decision. And we can, for example, see that certain areas are more highlighted than others, and perhaps that goes with the human intuition and creates more trust. TROND: You know, this topic is, for me, so fascinating because when we think about frontline workers or, indeed, engineers or quality managers on the shop floor, previously, they didn't have perhaps the tools available to open up the boxes, to open up the machines and look at the decisions that were being made. And, of course, that doesn't lead you to an enormous amount of confidence that what you're doing is good or bad or mediocre. You're not getting enough feedback. But it does seem to me that as machines and tools and algorithms on the shop floor become more and more complex, this is going to be a big effort. It doesn't sound very easy. And maybe you can characterize, you know, with the process today. These methods are just being applied on the shop floor. Do you have a sense that this general idea that things have to be explainable is a shared commodity on shop floors that are starting to use these techniques? Or would you say that it's enough of a challenge just to start experimenting with them, let alone trying to explain them to anybody around? I guess it's called a black box problem, right? JULIAN: Sure. I think in any use case where you have some kind of interaction between humans and AI, you've got to have explainability. It's going to be key. And there are also some less obvious use cases around explainable AI that I would also be happy to share. I think everywhere where you're going towards full automation, you perhaps don't need it, perhaps only if it's very high-stakes decisions being made. If you are rejecting products based on an AI that are very expensive, you might want to know why your line scrapped the products. In general, if you're going for automation, I would say explainability is nice to have. When we talk about augmentation, I think it's absolutely key. TROND: Yeah, it's absolutely clear. But we were talking before, and you were reminding me that in semiconductor production on an average production line, a large percentage of those components tend to be scrapped for quality reasons. And each of those components might be very, very expensive to manufacture. And it's a big problem. You have to recycle the part again, and they're made out of rare earth metals or whatnot. And it's a complicated thing, so it's not like you're just making wooden parts that you can just do over or plastic, and you can mold it again. These are like you said, they're expensive decisions that you're trusting machines to make. JULIAN: Yes. Talking about semiconductor industry, we have been also working on a different use case using explainable AI in semiconductors which I'm really excited about, and that is root cause analysis. In semiconductor manufacturing, as you mentioned, it's common that manufacturers throw away 15% to 20% of the chips they produce. We have car production lines that are standing still because of a chip shortage, so, obviously, this is a problem. What explainable AI can do here is we can try to model relationships in the manufacturing system to try to understand what causes these problems in the first place. So actually, when I was still a researcher back at ETH, I worked together with ABB semiconductors who exactly had this problem, so they had costly yield losses. And process engineers were struggling to find out where these losses were coming from. Because in semiconductor manufacturing, you often have hundreds of process steps, and each process, you can have even hundreds of process parameters such as temperatures and pressures. And you would like to know which of these process parameters do I need to adjust to avoid my yield losses. And if you have thousands, in this case, we had 3,600 different parameters that could have been suspects for yield losses. It's very hard to kind of track where yield losses are coming from. And the methods that are still used in industry are often based on linear methods. So we find this big effect, but we can't find the more hidden ones. And I think this is a very neat application of AI because you can use more complex models like neural networks or tree-based methods to model these relationships. So, in essence, we try to imitate the physical processes to learn the physical processes as they are. But since neural networks are very complex and we cannot really understand what they have been modeling, we need kind of explanations for that. And using these explanations, we can inform process engineers who are domain experts about what the model might have found. We're still acting upon correlations, not causation. But still, we can point towards certain areas that are interesting. And in the case of ABB, the AI guided the process engineers to suspicious processes, and the domain experts were able to come up with two improvement actions based on that input. Then they were able to reduce the scrap by more than 50% in one of their lines, which was, of course, substantial. And I think it's a very nice example of how humans and AI can collaborate and get more out of it. TROND: So, Julian, is that what augmentation of workers means to you? The augmented workforce is essentially a collaboration between man and machine in a deeper way than before? JULIAN: I think it's about expanding capabilities and getting teams of humans and machines that perform better and provide more value than either alone. I think that is what augmentation is about. MID-ROLL AD: In the new book from Wiley, Augmented Lean: A Human-Centric Framework for Managing Frontline Operations, serial startup founder Dr. Natan Linder and futurist podcaster Dr. Trond Arne Undheim deliver an urgent and incisive exploration of when, how, and why to augment your workforce with technology, and how to do it in a way that scales, maintains innovation, and allows the organization to thrive. The key thing is to prioritize humans over machines. Here's what Klaus Schwab, Executive Chairman of the World Economic Forum, says about the book: "Augmented Lean is an important puzzle piece in the fourth industrial revolution." Find out more on www.augmentedlean.com, and pick up the book in a bookstore near you. TROND: You ended up commercializing an idea around this. Tell me more about that and how that came about. JULIAN: Exactly. So at some point in my Ph.D., I decided I'm not going to become a professor; many PhDs have a similar experience. But I saw that 50% scrap reduction, for example, at one partner company that's quite substantial, and these tools kind of scale. And together with a friend of mine who did a Ph.D. in the same department, we decided let's found a company around this. So we founded a startup called EthonAI. And we are developing a software platform that helps manufacturers improve their quality management. So we offer five different products in different application areas, three computer vision products that help manufacturers in detecting defects, one product around process monitoring and anomaly detection, and one product for AI-based root cause analysis. So we cover this entire continuous improvement loop that manufacturers need, and we provide tools for that. And I think one thing that really stands out for us, and this is our key hypothesis, is that all of our products can be used by process engineers without writing a single line of code. We're not building fancy toolboxes for data scientists. We really build the tools for the people in the factory. We want to empower them to do their jobs better or help them do it better because we believe these are the people that drive the improvements on the shop floor. And those people should be the ones that use the tools. TROND: I'm just curious; in this process, you've talked to a lot of process engineers. How are they reacting to these new opportunities? Are they excited that they get to do some programming or do advanced analysis without getting deeply into software? Or are they a little bit weary that they have to still jump into a new domain? There's so much discussion these days about the need for re-skilling. How have you found them to react to these new changes? JULIAN: I think the experience is really mixed. Sometimes you see skepticism; sometimes, you see great excitement. I think at the end of the day, you need to solve problems. It's not about bringing AI to the factory. It's rather to solve the problems of the people that have worked in those factories. And if you have a tool that can be used very easily...so anyone that can operate a computer can operate our tools, and it solves problems. People are often happy to use it or mostly happy to use it. Of course, if you are coming in with this marketing thing of AI is going to change everything, then you experience more skepticism. So this is why we really talk about problems and not about the technology. Technology kind of is in the back end. And people don't care about how fancy your algorithms are; it has to work. What I think is so rewarding working on a startup in the manufacturing space is that the outcomes are so binary; it either works or it doesn't. And that's really cool to see in the physical world. Our entire team is working really hard to bring very useful tools to the market. TROND: You're a little bit of a hybrid yourself between a manufacturing engineer, basically, and now a little bit of a software engineer or at least an analytics perspective here with statistics and machine learning. Where do you find the expertise that you need at Ethon to move forward? Because it's a very rare thing still to combine knowledge of shop floor real-world challenges, systems that cannot fail, or at least when they fail, it has a bigger consequence than when a software needs to patch because the whole idea of why your software tentatively is in the shop floor is to reduce these kinds of stops and starts at production lines. How do you find people that really managed to combine the perspective of building software with this reality of how it's going to work in a physical environment? JULIAN: We have hired three kinds of people, first being brilliant scientists from the area of AI that have never been in a factory before but have been publishing at the highest level, NeurIPS, ICML, all those top outlets in the AI field. And they can really help to push the state of the art. The second category of people is process engineers. We've hired process engineers from companies that could be potential clients. They know about the problems and have been conducting all this data analysis in a quite manual way. So they're coming into our company to kind of guide us in building the product of their dreams. Then we have some people that have been working on the interface, such as Bernhard and myself. So Bernhard is the CTO in our company, people that have been on the interface between AI and manufacturing. So I think you really need basically experts and generalists in a company, and then it typically goes well. And then the other thing that you need is really customer-centricity. So you really need to be close to your customers and try to understand their pains. And you also need to bring the machine learning engineers to the factories so that they can see for themselves, and that's usually very helpful. TROND: What's your experience with frontline operators themselves? In the experiments that you've done with your Ph.D. and with your early products with Ethon, how are they reacting to these new opportunities for augmentation? JULIAN: I mean, typically, they're quite positive about it because it helps them in their jobs. But when we're talking about augmentation, it's not about replacing people; it's about helping them do things better. And often, they're quite critical about user interfaces, but you learn so much interacting with them, and you improve it. And then they get something that they really need. And the biggest learnings here have really been around how do you visualize or represent things or content to the workers? This is so crucial because, in factories, you have such a diverse workforce of people in their 60s who will retire soon. And you have young people who just started their first job and basically were raised with iPads. So it's really that's a challenge, but it's a great one to work on. TROND: Well, I guess that brings me to the important question that a lot of people want to answer about the factory of the future. There's fear about the factory of the future. There's this idea of a 24/7 automated factory, autonomous, working without humans. And then there's this idea that factories will look very different and people will do different things, but there are still people in there. And then there's anything in between. You could also imagine a world without factories but where industrial production happens somewhat distributed. Because I guess what a factory is, at the heart of it, is it's a mass of things, of people and machines concentrated in one location. JULIAN: Yes, it's a social, technical system. As I mentioned in the beginning, there is a lot of hype about AI, and these predictions are not new. This lights-out factory has been around for a very long time, and I still haven't seen it, at least on a productive level. You can also over-automate. Companies have seen that Tesla, for example, has cut back on automation. Elon Musk tweeted, "Humans are underrated." So I think in the future, people will still shape the image of factories. Of course, we will see more automation that is enabled through AI because, with AI, we can also now model stochastic processes. So we don't need deterministic outcomes like a robot that always picks the same things. We can have more self-organized, isolated systems. But I think it's really about isolated systems and not an entire factory that is operated by one single artificial brain. But I think the most intriguing use cases will be those where we augment humans rather than replacing them. So I think that's really where the magic happens. It's giving process engineers, for example, the tools to conduct more effective problem solving, finding things that were unknown to them previously, and couple that with their domain expertise. I think that's certainly something that we're going to see more. I don't think that AI will regulate production processes in a fully automated manner. At the end of the day, you always need the process and product expert that has intuition about the processes, creative problem-solving. So I think process engineers will always be around and be integral to any manufacturing system, but I think AI we will see more AI tools that enable these people and empower them. And I think this is an exciting development. TROND: Yeah, it's so interesting that sometimes I feel like these futuristic discussions fail to take into account innovation. So it's a very basic problem with this discussion because you're assuming that automation and factory production overall is all about squeezing out tiny, little efficiencies, and if that's all it is, then machines might be the better way to go. But if you're talking about incrementally improving and sometimes radically improving a process or changing even what you are producing based on feedback from a market and stuff like that, it would seem to me that we are quite far into the future before a socio-technical system like that with complete feedback, long supply chain, and understanding what all of these things mean and the decisions that go into it, and costs. And that all can be managed by one network algorithm. It's really a little bit hard to envision how those futurists really have been thinking about it, or maybe they were just considering isolated use of robots that looked very cool. JULIAN: The thing with AI is that AI is incredibly lazy; that's one of the problems. It always tries to learn shortcuts. And we need to understand the things that it learns. You always have the example of correlation and causation. And I think if you provide outputs from an AI to a human expert who can judge the validity of the results, that's where you can generate value. If an AI is supposed to make fully automated decisions, then I think it would relatively quickly turn out to be a mess because it might just be a correlation and not a causal relationship. There's always this famous example of shark attacks and ice cream consumption, both have a very high correlation, but it's not because sharks like ice cream. So I think it's very similar in manufacturing. When you produce stuff, you might find a correlation with a certain process parameter that can't causally have an effect on your quality, for example, or downtime. And if you would have a system that operates itself, it very likely would try to tweak that parameter with perhaps a bad outcome. So I think the human in the loop still remains crucial here. TROND: What excites you about the future of manufacturing? Everyone's always worried about manufacturing because it's such a big part of the economy. A lot of people lose their jobs if things go badly. And, historically, it's gone up and down. And maybe for a while, in some countries, it's gone mostly down, and it hasn't been the most exciting place to be. Are you excited about the future of manufacturing? JULIAN: Yes, I mean, definitely. I've always been, and I think, I will always be excited about manufacturing. And obviously, at EthonAI, we will also try to leave a mark on the industry. We are helping big companies to improve everything around quality but also helping them improve their CO2 footprint by reducing production waste. And this is something that really excites me to help these companies to provide useful tools and also see that these tools have an impact in the physical world at big corporations like Siemens. That's a very exciting place to be. I think we will see very, very interesting developments over the next couple of years. TROND: Well, Julian, it's exciting to jump in and hear a little bit about your world here. I certainly wish you best of luck with Ethon, and it was fascinating to hear. Thank you so much for sharing your perspective. JULIAN: Thank you very much, Trond. TROND: You have just listened to another episode of the Augmented Podcast with host Trond Arne Undheim. The topic was Explainability in AI. Our guest was Julian Senoner, CEO and Co-Founder of EthonAI. In this conversation, we talked about how to define explainable AI, its major applications, and its future. My takeaway is that explainability in AI, meaning knowing exactly what's going on with an algorithm, is very important for industry because its outputs must be understandable to the process engineers using it. The computer has not and will not use the product. Only a domain expert can recognize when the system is wrong, and that will be the case for a very long time in most production environments. Thanks for listening. If you liked the show, subscribe at augmentedpodcast.co or in your preferred podcast player, and rate us with five stars. If you liked this episode, you might also like Episode 103: Human-First AI with Christopher Nguyen. Hopefully, you'll find something awesome in these or in other episodes, and if so, do let us know. The Augmented Podcast is created in association with Tulip, the frontline operation platform that connects people, machines, devices, and systems. Tulip is democratizing technology and empowering those closest to operations to solve problems. Tulip is also hiring, and you can find Tulip at tulip.co. Please share this show with colleagues who care about where industrial tech is heading. You can find us on social media, and we are Augmented Pod on LinkedIn and Twitter and Augmented Podcast on Facebook and YouTube. Augmented — industrial conversations that matter. See you next time.Special Guest: Julian Senoner.